Artificial Intelligence (AI) broadly refers to technology that mimics human cognitive functions, defined as a machine's capability to imitate intelligent human behavior. The past decade in the AI field has been impressive, evolving from the You-Only-Look-Once (YOLO) object detection system in 2015 to the revolutionary language model GPT-4 release by OpenAI in 2023.
GPT-4’s capabilities have sparked widespread adoption, with 37% of businesses set to integrate AI into their operations to enhance efficiency and performance. Along with these advancements, a powerful subfield, Generative AI, has emerged to mark another key milestone in the evolution of this discipline. In a nutshell, Generative AI can create new data by learning from the existing data.
This article examines Generative AI and how it compares to Traditional (Analytical) AI, in terms of key features, benefits, and use cases to help technology leaders, enthusiasts, and business executives understand the key difference between the two concepts.
Table of Contents
What is Generative AI
What is Traditional (Analytical) AI
Generative AI vs Traditional (Analytical) AI: Head-to-head comparison
Generative AI vs Traditional AI: Use cases
Generative AI vs Traditional AI: How to choose the best fit for your business needs
Conclusion
What is Generative AI
Generative AI is a group of algorithms that can generate new information based on guiding prompts from the user. They rely on Unsupervised and Semi-supervised Machine Learning (ML) that allows computers to use information from text, audio, video files, images, and code—to produce new, original content that closely resembles the original.
Generative Adversarial Networks (GANs) and Variational Autoencoders (VAEs) were among the early examples of data generation using AI. However, the true GenAI revolution started with the invention of the Transformer architecture, which achieved remarkable results and powers key GenAI applications today.
At the current level of evolution, Generative AI brings impactful potential across different industries including Healthcare, Marketing, Software Development, Finance, and Entertainment applications, in terms of boosting efficiency and innovation.
Benefits of Generative AI
- Greater Creativity: Generative AI produces original texts, images, and music, enhancing creative processes with tools like ChatGPT for text generation, DALL-E for text-to-image creation, and other similar applications.
- Time and Cost Efficiency: GenAI can be used to automate time-consuming workflows in various industries. For instance, it automates content creation, reducing time and expenses compared to traditional methods.
- Personalization: GenAI models like ChatGPT and Gemini enable tailored content, enhancing engagement and user experience. These systems provide precise answers, eliminating the need to sift through numerous search pages for information.
Disadvantages of Generative AI
- Ethical Concerns: Since Generative AI models learn from existing work, this raises issues regarding Intellectual Property rights, copyright infringement, content ownership, and originality.
- Integration Complexity: Implementing GenAI involves technical challenges, requiring substantial expertise and resources. This complicates the integration of AI systems for many organizations.
- Lack of Control: Users may struggle with unpredictable outputs and hallucinations, in addition to the fact that Generative AI models often lack transparency, making it difficult to ensure consistent and reliable results.
What is Traditional (Analytical) AI?
Traditional AI (Weak AI or Narrow AI) is a branch of Artificial Intelligence that executes specific tasks using predefined algorithms and rules. These systems can learn from data and make decisions or predictions in unseen conditions.
Since, Traditional AI is trained to follow specific rules, it lacks the flexibility to adapt to new or complex situations. It cannot learn beyond its training data and does not innovate or generate new solutions.
Due to these limitations, its application is restricted, and industries and businesses often use Traditional AI for specific tasks such as data analysis, speech recognition, image and text classification, object detection, fraud detection, and customer service automation.
Benefits of Traditional AI
- Resource-efficient: Traditional AI systems are designed for specific tasks, optimizing resource use and reducing costs compared to broader Artificial Intelligence models.
- High Accuracy (Specific Domains): Traditional AI can achieve high accuracy in well-defined domains, leveraging specialized algorithms and training data to excel in tasks like image recognition, diagnostics, and data analysis.
- Transparency: Traditional AI models are often easier to interpret and understand, enhancing transparency and making it simpler to audit and validate their decisions.
Disadvantages of Traditional AI
- Limited Adaptability: Traditional AI systems are tailored to specific tasks and struggle to adapt to new, unforeseen challenges or domains without extensive reprogramming.
- Human Dependent: These systems often require significant human oversight and intervention, particularly for tasks involving complex decision-making or when faced with ambiguous situations.
- Limited Scope: Traditional AI excels in defined domains but lacks the generalization capabilities needed for broader cognitive tasks or interdisciplinary applications.
Generative AI vs Traditional (Analytical) AI: Head-to-Head Comparison
This section examines Generative AI vs Traditional (Analytical) AI by exploring their primary functions, training methodologies, data types they handle, and their respective application areas.
Generative AI vs Traditional AI: Use Cases
Real-world Applications of Generative AI
GenAI has a creative edge over Traditional (Analytical) AI which allows it to create new data from its learning. For this reason, some of the distinct Generative AI use cases include:
- Text-to-Image Generation: Models like Dall-E and Stable Diffusion can understand text prompts and generate images from the provided instructions. These models are useful for developing creative content such as animated characters, logos, and digital art.
- Intelligent Chatbots: GenAI has pioneered sequence-to-sequence modeling and GenAI-powered chatbots that can conduct human-like conversations. Applications like ChatGPT and Gemini can understand human queries and develop natural and factually accurate responses.
- Text Summarizer: GenAI bots can understand key elements from long blocks of text and use their semantic understanding and the key ideas to generate summaries for the text document.
Real-world Applications of Traditional (Analytical) AI
On the other hand, some of the key Analytical AI use cases that have been implemented across various industries include;
- Demand Forecasting: Forecasting algorithms are used to predict how the sales and demand of a product will vary in the future. This insight is used for inventory management, planning the production, marketing, and sales workflows in the Retail sector, for instance.
- Customer Segmentation: Clustering algorithms are used to create segments of the customer base. Each cluster represents customers from different demographics and purchase patterns. These allow companies to plan their marketing campaigns more efficiently.
- Credit Scoring: Classification algorithms are used to process a person’s personal and financial information and assign them a credit score for Financial service providers. This automates the manual process, improving work efficiency.
Generative AI vs Traditional AI: Shared Use Cases
Although GenAI and Traditional AI have certain distinctions, they also share several key application areas when it comes to solving real-world problems. Some of them include:
Fraud Detection: Traditional (Analytical) AI typically uses historical data to train models for detecting fraudulent transactions, while Generative AI, such as GANs, generate synthetic data to enhance detection accuracy and improve the robustness of fraud models by increasing the number of data records used to train fraud detection models and the variety and sophistication of examples.
Spam Classification: Both AI types are effective in combating unwanted emails. Traditional AI uses supervised learning on labeled datasets to distinguish between spam and legitimate emails. At the same time, Generative AI can analyze email content using Natural Language Processing (NLP) models to detect spam. Primarily, it leverages generative techniques to understand and classify email content accurately without extensive labeled datasets.
Image to Text & OCR: Both Generative and Traditional AI can convert images to text. Optical Character Recognition (OCR) enables machines to read text in images, allowing processing by programs and scripts. Traditional OCR models include Tesseract and EasyOCR, while Generative models, such as GPT-4 Vision and Gemini Pro 1.5, enhance this capability.
Generative AI vs Traditional AI: How to Choose the Best Fit For Your Business Needs
When deciding between GenAI and Traditional AI, several key considerations should be evaluated to ensure the chosen solution aligns with your business needs. These factors include; data availability, resource availability, and the need for model explainability.
Data Availability:
- Generative AI benefits from large, diverse datasets, which enhance the training of models with Large Language Models (LLMs). This makes them generalize over a wide range of problems.
- Traditional AI can operate effectively with smaller, structured datasets suitable for classification or regression tasks.
Resource Availability:
- Generative AI models, especially larger ones, require substantial computational resources and time for training. Such models, therefore, are more powerful in generating content.
- Traditional AI models are generally less resource-intensive, allowing quicker development and deployment.
Explainability:
- Traditional AI models often offer better explainability and transparency, facilitating easier understanding and interpretation of results.
- Generative AI models, while powerful, may require additional techniques to enhance interpretability and ensure transparency and trust.
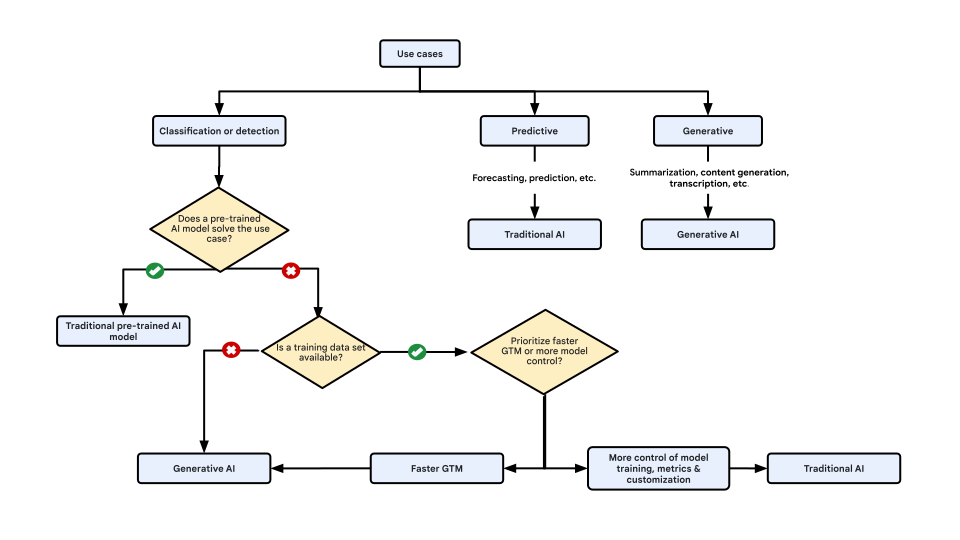
Conclusion
In the article, we discussed the evolution of Artificial Intelligence and highlighted its division into two main categories: Traditional AI and Generative AI. GenAI capabilities are impressive, but the need for Traditional AI remains. Traditional AI is efficient and accurate for specific tasks but lacks adaptability and requires significant human oversight. Generative AI boosts creativity and personalization but raises ethical concerns and can produce unpredictable outputs.
Noting these distinctions can help technology leaders and corporate executives make informed decisions and choose the most appropriate technology for their specific business challenges.
You may also be interested in:
AI Readiness Assessment: The Complete Guide for Companies
How to Achieve SOC 2 Compliance in AWS Cloud Environments
Monolithic vs Microservices Architecture: Key Differences
Bluelight Consulting is a Certified SOC 2 Compliant Company
IT Outsourcing: Key Services, Engagement Models & Benefits
Web Development Services: The Complete Guide for Businesses
More cost-effective than hiring in-house, with Nearshore Boost, our nearshore software development service, you can ensure your business stays competitive with an expanded team and a bigger global presence, you can be flexible as you respond to your customers’ needs.
Learn more about our services by booking a free consultation with us today!