Data is the foundation of the new technological era that businesses are navigating today. Many companies rely on data technologies for their operations, yet still struggle to become truly data-driven or fail to extract and build value from their own data.
The book Fundamentals of Data Engineering [O'Reilly Media; 2022] introduces three levels of Data Maturity that companies can aim for. Each level presents its challenges and benefits, but they all share a common goal: to find a balance between delivering value to the business and evolving the data ecosystem.
This challenge often arises because building a well-integrated data ecosystem aligned with the business core is required before any benefits can be realized. This data ecosystem can be divided into three levels of maturity, which this article explores in detail.
Table of Contents
The 3 Key Levels of Data Maturity in Companies
Case Studies of Data Maturity Levels in Companies: Top 5 Examples to Learn From
How to Identify Lack of Data Maturity in Companies
A Practical Approach to Data Maturity Gap Analysis for Companies
What is a Data Ecosystem
A data ecosystem is the interconnected framework of tools, technologies, processes, and people that enable a company to collect, store, process, and utilize data effectively. It includes;
- Infrastructure - Databases, cloud storage, network
- Governance - policies, compliance, data lineage
- Workflows - ETL, ELT, analytics pipelines
What is Data Maturity?
Data maturity measures how advanced a company’s data process is. A high level of data maturity is the stage where data is deeply woven into the fabric of an organization and has become incorporated into every decision that an organization makes.
The 3 Key Data Maturity Levels in Companies
Level 1: Starting with Data
At this data maturity stage, companies are in the early stage of employing data in their operations and most decisions are made based on the experience and instincts of Business Analysts.
A common challenge here is the lack of clear goals. Even if there is a desire to leverage data, it can be difficult to determine where to start and which technologies to adopt. Furthermore, early data initiatives are often driven by a few key individuals who lead internal transformation efforts.
These leaders need clarity on how to effectively guide this transformation. For this reason, their task is to identify stakeholders who can benefit from data initiatives and develop a plan that integrates all parties.
The primary goal at this stage is to plant the seeds of a data culture, encouraging decision-making based on data, and demonstrating its value. However, as with any cultural shift, there may be resistance, and overcoming this requires addressing key pain points. To demonstrate the value of data, early wins are crucial. Typical initiatives at this stage include:
- Automating existing metrics: Many companies already track operational metrics. Automating data collection for these metrics and presenting them in real-time dashboards can reduce manual effort and provide immediate insights.
- Establishing basic data analysis: Creating initial reports and dashboards that describe the business using its own data helps highlight sensitive areas and gaps, paving the way for new initiatives.
- Identifying data silos: Start by cataloging all relevant data sources within the company to integrate them effectively.
At this stage, it is common for roles to be not well defined, which calls for technical personnel to adopt a generalist approach when developing data products. Additionally, accumulating technical debt is common but acceptable if managed wisely.
Overall, the key focus should be on delivering value.
Level 2: Scaling with Data
Once a company has basic data processes in place and is making some data-driven decisions, the next step is to scale these efforts.
At this data maturity level, processes become more complex, and Data Analysts begin taking the initiative to identify new projects, adding them to the data team’s backlog. This scaling phase is critical and must be managed carefully to avoid growing pains.
Here, roles become more specialized, with clear distinctions between Data Engineers and Data Analysts. On one hand, Data analysts work closely with Business Analysts (and sometimes overlap their roles) to identify opportunities that drive business value.
Meanwhile, Data Engineers tackle technical challenges, ensuring data quality and scalability on the infrastructure and processes as the work volume and complexity grows.
Every decision—whether technical or strategic—should align with the company's goals. A common bottleneck to highlight at this stage is the absence of clear objectives, both for people and processes. Nonetheless, it's essential to maintain a clear direction and a focus on delivering business value.
It is worth noting that Data pioneers play a key role in promoting how to consume and leverage data across the organization while specializing in their roles and optimizing the data lifecycle at the same time.
Level 3: Leading with Data
Companies at this stage of evolution are considered mature in their data products and processes. They are often industry leaders, setting trends for others to follow.
Automation is standard across all layers, with self-service analytics solutions widely available. Moreover, all data is cataloged and traceable, and integrating new data sources into the company’s data lifecycle is a routine task. Last but not least, compliance and quality controls are robust and well-established.
However, the challenges at this level often stem from complexities introduced during the scaling phase. With increasing complexity in environments, products, and processes, there’s a risk of losing focus on what truly delivers value.
It’s easy to get sidetracked by exciting but non-essential projects. The real danger here is falling for technological hype and incorporating unnecessary tools that add complexity without value. Decision-makers need the sensitivity and wisdom to prioritize what genuinely contributes to business goals.
Leadership at this data maturity stage involves ensuring all teams are aligned with the company's goals, facilitating cross-functional collaboration, and proactively managing the business’s strategic direction.
Case Studies of Data Maturity Levels in Companies: Top 5 Examples to Learn From
Level 1: Starting with Data - Netflix in its early days
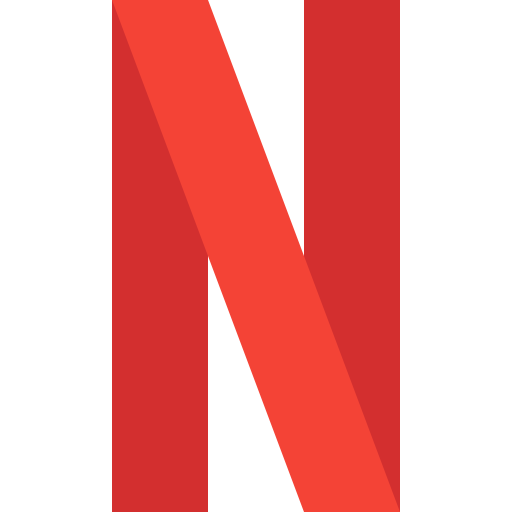
In its early days as a DVD rental company, Netflix relied on limited data to understand customer preferences. Data-driven efforts were primarily focused on tracking operational metrics, such as DVD rental turnaround times. Their use of data was rudimentary but set the foundation for their later transformation into a data-driven streaming giant.
Level 2: Scaling with Data - Uber and Airbnb Case Studies
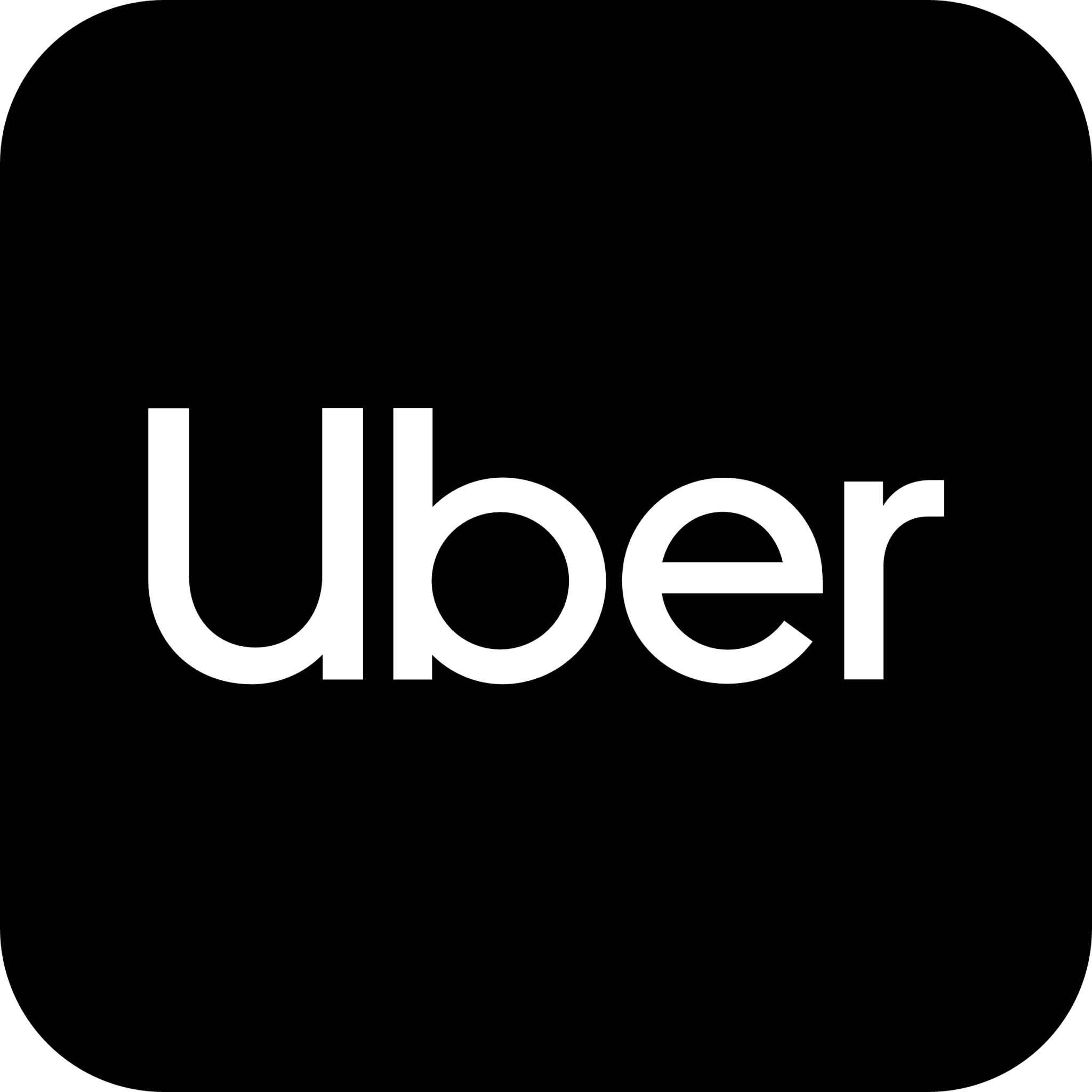
As Uber expanded globally, it needed to scale its data systems to handle increasing complexity. The company optimized routing for drivers, implemented dynamic pricing algorithms, and enhanced customer experience through data. Specialized data teams were built to tackle specific challenges, such as data integration from multiple cities.

On the other hand, Airbnb scaled its data infrastructure to better understand user behavior and optimize the platform experience for hosts and guests. This scaling allowed them to enhance product offerings and refine their decision-making processes.
Level 3: Leading with Data - The Amazon and Google Case Studies
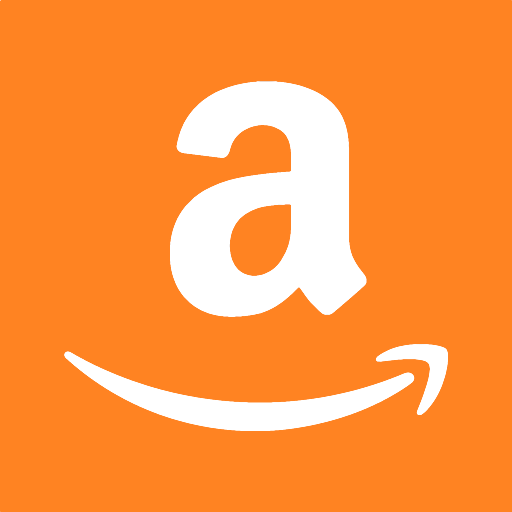
Amazon is a leader in leveraging data across every aspect of its business. From its highly automated supply chain logistics to personalized recommendations, data drives all decisions.
The company has established self-service analytics for employees, robust data cataloging, and seamless integration of new data sources. It exemplifies a company at the forefront of data maturity, navigating innovation without losing sight of its business goals.
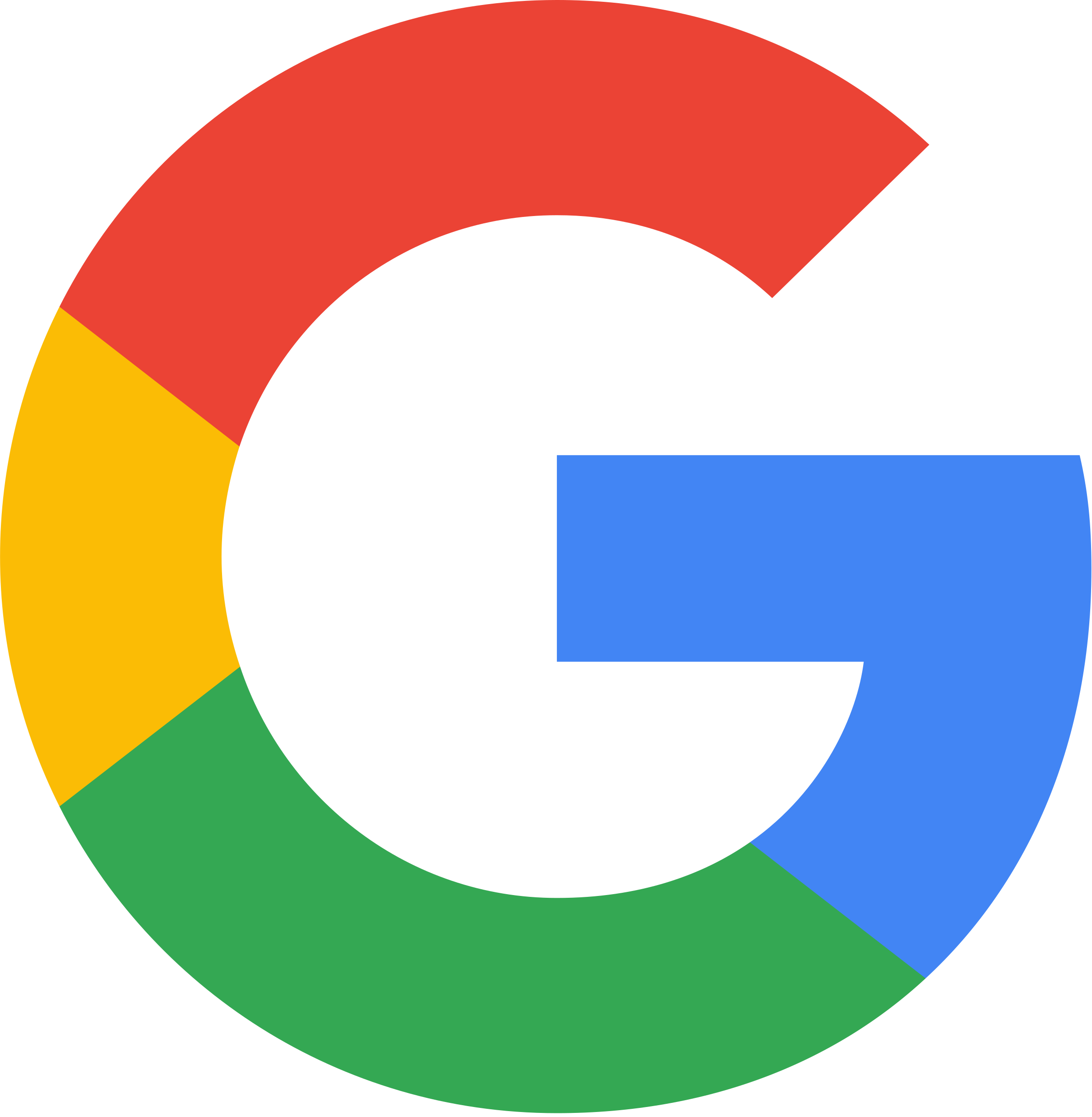
On its part, Google operates at the pinnacle of data maturity, using data systems that power its core businesses, such as Search, Ads, and Cloud solutions. Google’s automated and scalable processes set the industry standard.
How to Identify Lack of Data Maturity in Companies
Recognizing a lack of data maturity within your organization is the first step toward improvement. Identifying gaps allows you to address weaknesses systematically and advance your company’s data capabilities.
Here’s a guide on how to spot the absence of data maturity best practices at each level:
1. Limited Data Usage and Decision-Making
At the earliest stages of data maturity, companies often struggle to incorporate data into their decision-making processes. Key warning signs include:
- Gut-driven decisions: Most decisions are based on intuition or the experience of leaders, with little or no reference to data.
- No central data repository: Data is scattered across spreadsheets, email chains, and siloed systems, making it difficult to consolidate or analyze.
- Resistance to change: Teams may question the value of data-driven approaches or feel overwhelmed by the learning curve required to adopt them.
- Ad hoc reporting: Any analysis performed is typically reactive, generated in response to immediate needs rather than proactively aligned with business goals.
2. Inefficiencies in Scaling Data Efforts
Organizations attempting to scale their data operations may encounter bottlenecks that hinder progress. Indicators of this include:
- Undefined roles: The lack of clear distinctions between Data Engineers, Analysts, and business stakeholders results in inefficiencies or duplicated efforts.
- Backlog overflow: The data team’s workload becomes unmanageable due to increasing demands for reports, dashboards, and analytics, leading to delays and missed opportunities.
- Data quality issues: Poor data governance leads to inconsistencies, inaccuracies, and gaps in the data being analyzed.
- Fragmented tools and processes: Teams adopt disparate technologies without clear integration, creating friction and complexity.
3. Misalignment at High Levels of Complexity
Even companies implementing the best data governance practices can suffer from gaps in maturity, particularly if their operations become too complex to manage effectively. Common red flags include:
- Overemphasis on technology: Investing in shiny new tools without clear alignment with business needs can distract teams and waste resources.
- Siloed data insights: Advanced analytics capabilities may exist within isolated departments, but insights are not effectively shared or integrated across the organization.
- Diminished focus on value: Data teams may get caught up in technical achievements or experimental projects that don’t contribute meaningfully to business objectives.
- Compliance risks: As processes and systems grow more sophisticated, neglecting robust data governance can lead to non-compliance with legal and regulatory requirements.
A Practical Approach to Data Maturity Gap Analysis for Companies
To pinpoint areas of improvement, consider the following steps:
- Conduct a self-assessment: Evaluate your company’s data practices against the key characteristics of each maturity level. Identify whether decision-making is data-driven, where bottlenecks exist, and how well data supports business goals.
- Engage stakeholders: Gather input from leaders and teams across departments to understand their challenges and expectations regarding data usage.
- Audit your tools and processes: Inventory your current data technologies and workflows to identify redundancies, inefficiencies, or gaps in integration.
- Assess data governance: Review how data is cataloged, maintained, and secured. Also, pay attention to compliance requirements and the scalability of existing systems.
- Prioritize actions: Focus first on the most significant barriers to progress. Quick wins, such as automating manual reporting or improving data quality, can build momentum for further initiatives.
By identifying and addressing gaps in data maturity, your organization can pave the way for stronger decision-making, enhanced scalability, and ultimately, a competitive advantage in the market.
Conclusion
The three levels outlined in this article serve as a guide to identify areas for improvement and to provide clarity on your company's data maturity. However, organizations can be complex, with different business areas often falling into different levels of data maturity.
Rather than forcing your company into a predefined category, it is advisable to understand its unique business, operations, as well as data processes and aim to drive continuous improvement.
You may also be interested in:
Best C++ IDEs: A Review of the Top 6 in Detail
How to Build an Effective AI Strategy for Your Business
Generative AI vs Traditional AI: The Complete Comparison
AI Readiness Assessment: The Complete Guide for Companies
How to Achieve SOC 2 Compliance in AWS Cloud Environments
More cost-effective than hiring in-house, with Nearshore Boost, our nearshore software development service, you can ensure your business stays competitive with an expanded team and a bigger global presence, you can be flexible as you respond to your customers’ needs.
Learn more about our services by booking a free consultation with us today!